The house I grew up in was so close to my high school that if I poked my head out of our bathroom window on the top floor, I could actually see my fellow students scampering around the yard during their PE classes. On occasion I’d daydream about how much time I might save if I could somehow string a zipline between the two endpoints and glide onto campus every morning.
Not that my three-minute commute was at all unmanageable. Because our house and the school occupied the same Brooklyn block, I could get from breakfast to Biology simply by tracing the perimeter of our shared rectangle, no crosswalks required. This level of self-containment comforted me — I liked knowing that if all of the surrounding streets spontaneously sunk to the center of the earth, separating each block into its own island, well then I could still get to school everyday, and even pick up a croissant from Ozzie’s along the way.
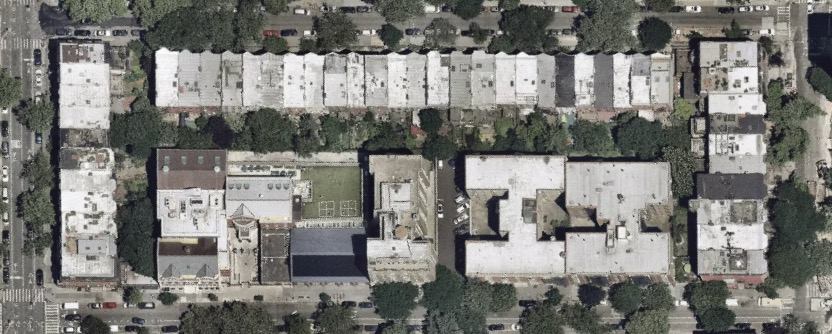
I’ve carried this appreciation for proximity into my adulthood, during which I’ve lived in a handful of apartments across various Manhattan neighborhoods. There’s something extra satisfying about being able to procure a coffee, a beer, a roll of toilet paper without straying from your block of origin. And so I wondered: which block in New York City has the most resources? If you wanted to get the most out of life without ever crossing the street, where should you live? In a city more or less devoid of traditional malls, can we recreate one in the aggregate on a single block?
To answer this question we need to first divide the city up into the blocks created by its signature grid pattern. To do so, we take a shapefile of New York City streets (sourced from NY.gov) and remove that area from a larger shapefile of the five boroughs (sourced from NYC OpenData). It’s kind of like the streets are a giant cookie cutter that we’re using to press out individual biscuits from the city’s terrain.
Next, we need to list and locate as many of the city’s businesses – restaurants, bars, supermarkets, gyms, laundromats, etc. – as we can. Thankfully, the folks at Open Street Maps provide their Overpass API, which, given a geographical bounding box, will tell you exactly what they can find within it, down to park benches and bike racks. (It should be noted that there appears to be some missing locations from this dataset, especially as you stray further from Manhattan; while I was able to verify the map’s accuracy in regions close to my Upper East Side apartment, I couldn’t find some of my go-to lunch spots near my office in the Bronx.)
Here is where the the analysis tips over into unavoidable subjectivity. How do we define a utility function to grade the resources available at each city block? As far as I’m concerned, a Chipotle, a Dunkin’, two bars, a laundromat, and a gym would more than suffice (if you can’t tell, I’m a man of culture). But my mom would probably like a yoga studio, a sushi restaurant, and a flower store. And beyond preference, there’s the question of saturation – at what point is the marginal value of another restaurant eclipsed by something less exciting, like a post office?
Understanding that I can’t please everybody (or maybe even anybody), I’ve assigned the following points for each category as displayed in the table below. Blocks accumulate points per location
for each instance of a particular category, but are also capped at max points
in that category – so that, for example, seven restaurants would be no more valuable than six. The most important rows are the high frequency ones, like cafes, bars, and supermarkets, but for the sake of thoroughness I evaluated the utility of every last category available. Yes, I think a “swingerclub” is worth a point (and probably more, to be quite honest).
category | frequency | points per location | max points |
---|---|---|---|
restaurant | 6673 | 1 | 6 |
fast food | 3115 | 1 | 6 |
cafe | 2021 | 1 | 4 |
convenience | 1656 | 3 | 9 |
place of worship | 1614 | 1 | 2 |
bar | 1436 | 1 | 4 |
clothes | 1431 | 2 | 4 |
school | 1392 | 0 | 0 |
hairdresser | 1152 | 2 | 4 |
beauty | 1083 | 2 | 4 |
pharmacy | 991 | 3 | 6 |
bank | 990 | 2 | 4 |
bicycle rental | 843 | 0 | 0 |
supermarket | 798 | 4 | 12 |
alcohol | 689 | 2 | 4 |
deli | 666 | 2 | 6 |
laundry | 604 | 2 | 4 |
bakery | 591 | 1 | 2 |
mobile phone | 491 | 0 | 0 |
fitness centre | 478 | 4 | 8 |
dry cleaning | 419 | 2 | 4 |
dentist | 411 | 0 | 0 |
clinic | 376 | 3 | 6 |
doctors | 363 | 0 | 0 |
variety store | 349 | 2 | 4 |
park | 331 | 4 | 8 |
jewelry | 291 | 1 | 2 |
car repair | 288 | 0 | 0 |
post box | 287 | 1 | 1 |
optician | 281 | 0 | 0 |
fuel | 265 | 0 | 0 |
ice cream | 243 | 2 | 4 |
post office | 236 | 1 | 1 |
fire station | 235 | 3 | 3 |
shoes | 235 | 2 | 4 |
gift | 234 | 2 | 4 |
furniture | 203 | 1 | 1 |
tobacco | 196 | 1 | 2 |
florist | 175 | 1 | 1 |
hardware | 165 | 2 | 2 |
kindergarten | 157 | 0 | 0 |
bicycle | 156 | 0 | 0 |
department store | 153 | 2 | 4 |
massage | 151 | 3 | 3 |
theatre | 150 | 3 | 6 |
cosmetics | 144 | 2 | 4 |
greengrocer | 140 | 2 | 4 |
childcare | 136 | 4 | 4 |
books | 134 | 2 | 4 |
social facility | 133 | 0 | 0 |
atm | 118 | 1 | 2 |
sports centre | 117 | 3 | 6 |
pet | 116 | 0 | 0 |
butcher | 110 | 2 | 4 |
car | 108 | 0 | 0 |
veterinary | 104 | 3 | 3 |
library | 102 | 3 | 3 |
tattoo | 97 | 0 | 0 |
arts centre | 95 | 2 | 4 |
cannabis | 89 | 2 | 4 |
nightclub | 89 | 2 | 4 |
electronics | 88 | 1 | 2 |
police | 88 | 3 | 3 |
car rental | 83 | 0 | 0 |
community centre | 75 | 3 | 3 |
events venue | 72 | 3 | 3 |
sports | 71 | 3 | 3 |
telephone | 71 | 1 | 1 |
nutrition supplements | 70 | 2 | 4 |
public bookcase | 70 | 1 | 1 |
copyshop | 68 | 2 | 2 |
yes | 68 | 0 | 0 |
car parts | 66 | 0 | 0 |
confectionery | 64 | 2 | 4 |
funeral directors | 64 | 0 | 0 |
marketplace | 64 | 3 | 3 |
stationery | 62 | 2 | 2 |
garden | 61 | 3 | 3 |
seafood | 60 | 1 | 3 |
studio | 60 | 2 | 2 |
houseware | 58 | 2 | 2 |
cinema | 56 | 3 | 6 |
dance | 56 | 3 | 6 |
parking | 56 | 0 | 0 |
toys | 55 | 2 | 2 |
trade | 55 | 2 | 2 |
tyres | 55 | 0 | 0 |
charging station | 52 | 0 | 0 |
craft | 52 | 2 | 2 |
art | 47 | 2 | 2 |
ferry terminal | 47 | 0 | 0 |
kitchen | 47 | 2 | 2 |
newsagent | 46 | 1 | 1 |
bed | 44 | 0 | 0 |
e-cigarette | 44 | 1 | 1 |
paint | 42 | 1 | 1 |
pet grooming | 41 | 2 | 2 |
playground | 41 | 3 | 3 |
second hand | 41 | 2 | 4 |
wine | 40 | 2 | 4 |
party | 39 | 2 | 2 |
taxi | 39 | 0 | 0 |
car wash | 38 | 0 | 0 |
chocolate | 38 | 1 | 2 |
driving school | 38 | 0 | 0 |
video games | 38 | 2 | 2 |
beverages | 37 | 1 | 3 |
hospital | 37 | 4 | 4 |
parking entrance | 36 | 0 | 0 |
storage rental | 36 | 0 | 0 |
tailor | 36 | 2 | 4 |
travel agency | 35 | 0 | 0 |
wholesale | 35 | 2 | 2 |
doityourself | 34 | 1 | 1 |
pawnbroker | 34 | 2 | 2 |
perfumery | 34 | 2 | 2 |
charity | 33 | 1 | 1 |
frame | 33 | 1 | 1 |
interior decoration | 33 | 2 | 2 |
music | 33 | 2 | 4 |
antiques | 32 | 2 | 4 |
fashion accessories | 32 | 1 | 2 |
appliance | 31 | 2 | 4 |
college | 31 | 0 | 0 |
locksmith | 31 | 1 | 1 |
animal boarding | 30 | 2 | 4 |
university | 30 | 0 | 0 |
fabric | 29 | 1 | 1 |
music school | 28 | 0 | 0 |
drinking water | 27 | 0 | 0 |
bag | 26 | 0 | 0 |
food court | 26 | 3 | 3 |
coffee | 25 | 1 | 3 |
herbalist | 25 | 1 | 1 |
religion | 25 | 1 | 1 |
computer | 24 | 2 | 2 |
medical supply | 23 | 2 | 2 |
musical instrument | 23 | 2 | 2 |
carpet | 21 | 0 | 0 |
courthouse | 21 | 0 | 0 |
erotic | 21 | 2 | 2 |
photo | 21 | 2 | 2 |
bus station | 20 | 0 | 0 |
pitch | 20 | 0 | 0 |
garden centre | 19 | 2 | 4 |
tea | 19 | 1 | 3 |
grave yard | 18 | 0 | 0 |
lighting | 18 | 1 | 1 |
baby goods | 17 | 1 | 1 |
health food | 17 | 1 | 2 |
watches | 17 | 1 | 1 |
tiles | 16 | 1 | 1 |
bureau de change | 15 | 1 | 1 |
general | 15 | 1 | 1 |
social centre | 15 | 1 | 1 |
townhall | 15 | 1 | 1 |
hairdresser supply | 14 | 1 | 1 |
bbq | 13 | 1 | 2 |
marina | 13 | 0 | 0 |
stripclub | 13 | 2 | 4 |
vending machine | 13 | 1 | 1 |
cheese | 12 | 2 | 4 |
fountain | 12 | 0 | 0 |
motorcycle | 12 | 0 | 0 |
outdoor | 12 | 0 | 0 |
sewing | 12 | 1 | 1 |
toilets | 12 | 0 | 0 |
window blind | 12 | 1 | 1 |
money lender | 11 | 1 | 1 |
telecommunication | 11 | 1 | 1 |
ticket | 11 | 0 | 0 |
bathroom furnishing | 10 | 1 | 1 |
bicycle parking | 9 | 0 | 0 |
boat rental | 9 | 0 | 0 |
clock | 9 | 0 | 0 |
farm | 9 | 0 | 0 |
flooring | 9 | 1 | 1 |
language school | 9 | 0 | 0 |
vacant | 9 | 0 | 0 |
animal shelter | 8 | 1 | 1 |
biergarten | 8 | 1 | 3 |
collector | 8 | 1 | 1 |
escape game | 8 | 0 | 0 |
hackerspace | 8 | 0 | 0 |
shelter | 8 | 0 | 0 |
doors | 7 | 0 | 0 |
leather | 7 | 0 | 0 |
mall | 7 | 4 | 4 |
miniature golf | 7 | 1 | 1 |
vacuum cleaner | 7 | 0 | 0 |
games | 6 | 1 | 1 |
parcel locker | 6 | 0 | 0 |
slipway | 6 | 0 | 0 |
video | 6 | 1 | 1 |
car sharing | 5 | 0 | 0 |
dog park | 5 | 3 | 3 |
hearing aids | 5 | 0 | 0 |
ice rink | 5 | 0 | 0 |
internet cafe | 5 | 1 | 2 |
swimming pool | 5 | 3 | 3 |
bench | 4 | 0 | 0 |
camera | 4 | 1 | 1 |
curtain | 4 | 1 | 1 |
electrical | 4 | 1 | 1 |
give box | 4 | 0 | 0 |
military surplus | 4 | 1 | 1 |
money transfer | 4 | 1 | 1 |
music venue | 4 | 3 | 3 |
nature reserve | 4 | 3 | 3 |
nursing home | 4 | 0 | 0 |
public bath | 4 | 0 | 0 |
recycling | 4 | 0 | 0 |
training | 4 | 0 | 0 |
amusement arcade | 3 | 2 | 4 |
fitness station | 3 | 2 | 4 |
hifi | 3 | 0 | 0 |
pasta | 3 | 1 | 1 |
public building | 3 | 0 | 0 |
security | 3 | 0 | 0 |
trophy | 3 | 0 | 0 |
anime | 2 | 1 | 1 |
boat | 2 | 0 | 0 |
boutique | 2 | 2 | 4 |
common | 2 | 0 | 0 |
fishing | 2 | 0 | 0 |
fortune teller | 2 | 1 | 1 |
frozen food | 2 | 1 | 2 |
gambling | 2 | 1 | 1 |
glaziery | 2 | 0 | 0 |
monastery | 2 | 0 | 0 |
motorcycle parking | 2 | 0 | 0 |
prison | 2 | 0 | 0 |
research institute | 2 | 1 | 1 |
spices | 2 | 1 | 1 |
waste basket | 2 | 0 | 0 |
weapons | 2 | 1 | 1 |
beach resort | 1 | 0 | 0 |
bookmaker | 1 | 0 | 0 |
brewing supplies | 1 | 0 | 0 |
cafe;bar | 1 | 1 | 1 |
casino | 1 | 2 | 2 |
conference centre | 1 | 1 | 1 |
dojo | 1 | 0 | 0 |
driver training | 1 | 0 | 0 |
graphic design | 1 | 1 | 1 |
household linen | 1 | 1 | 1 |
lottery | 1 | 1 | 1 |
outpost | 1 | 0 | 0 |
pest control | 1 | 0 | 0 |
planetarium | 1 | 0 | 0 |
post depot | 1 | 0 | 0 |
ranger station | 1 | 0 | 0 |
spa | 1 | 3 | 3 |
swingerclub | 1 | 1 | 1 |
vehicle inspection | 1 | 0 | 0 |
waste transfer station | 1 | 0 | 0 |
wool | 1 | 0 | 0 |
So now that we have a map and a scoring system, which blocks provide the most convenience to their inhabitants? If you limit your search to areas smaller than 200,000 square feet, so as to avoid useless answers like LaGuardia Airport, two Manhattan blocks surpass fifty points and take our top spots. In second place is the block between 32nd and 33rd Streets and 5th and Broadway avenues in the Koreatown neighborhood of Manhattan. As demonstrated below, this block has over a dozen food options – many of them Korean barbecue – and also cafes, convenience stores, four bars, both a Walgreens and a CVS, a bank, a bookstore, and a massage parlor. So packed with stores and restaurants is the segment along 32nd street that it’s often referred to as the heart of Koreatown and has even been nicknamed “Korea Way”.
Koreatown is more of a commercial area than a residential one though, so it’s harder to imagine actually living on top of this stretch of businesses. More intriguing is the first place finisher located in the East Village, a neighborhood overflowing with so much culture that young New Yorkers are willing to squeeze themselves into small, pricey, oddly-shaped apartments just to experience it. This block is surrounded by 9th St, 10th St, 1st Avenue, and 2nd Avenue and it too has plenty of dining options, but also a grocery store, a nail salon, a laundromat, a dentist, an optician, and even a butcher.
Are this block’s inhabitants aware that they reside atop arguably the peak of New York City convenience? To answer that question, I had to engage in some field work, a rare task for the sedentary blogger. So on a cold Monday night in January, I posted up on 10th St and 2nd Avenue and asked some of the local residents if they had considered their block’s abundant resources and taken advantage of them accordingly.
One man noted how easy it was to “go for a bite or a drink,” while another praised the convenience store that was just around the corner. Two people listed Madame Vo, a Vietnamese restaurant on 10th St, as a frequent destination. But overall they didn’t share the appreciation I had for their block’s top status, and were quick to list businesses that fell outside of their atomic rectangle, violating the constraints of my optimization. It appears that an obsession with extreme proximity might be reserved for people lucky enough to have lived around the corner from school.
In any case, below are the top ten blocks in our dataset with additional details available if you expand the locations
header:
borough | streets | points | ||||||||||||||||||||||||||||||||||||||||||||||||
---|---|---|---|---|---|---|---|---|---|---|---|---|---|---|---|---|---|---|---|---|---|---|---|---|---|---|---|---|---|---|---|---|---|---|---|---|---|---|---|---|---|---|---|---|---|---|---|---|---|---|
Manhattan | E 10th St, E 9th St, 1st Ave, 2nd Ave |
54
locations
|
||||||||||||||||||||||||||||||||||||||||||||||||
Manhattan | Broadway, W 32nd St, W 33rd St, 5th Ave |
52
locations
|
||||||||||||||||||||||||||||||||||||||||||||||||
Queens | Horace Harding Expy, Springfield Blvd, NA |
49
locations
|
||||||||||||||||||||||||||||||||||||||||||||||||
Queens | 70th Rd, Austin St, Queens Blvd, 71st Ave |
48
locations
|
||||||||||||||||||||||||||||||||||||||||||||||||
Manhattan | Cooper Sq, E 7th St, Saint Marks Pl, Taras Shevchenko Pl, 2nd Ave |
47
locations
|
||||||||||||||||||||||||||||||||||||||||||||||||
Brooklyn | Fulton St, Jay St, Lawrence St, Willoughby St |
46
locations
|
||||||||||||||||||||||||||||||||||||||||||||||||
Queens | 40th Rd, Main St, Prince St, Roosevelt Ave |
45
locations
|
||||||||||||||||||||||||||||||||||||||||||||||||
Manhattan | Bowery, Chrystie St, Grand St, Hester St |
44
locations
|
||||||||||||||||||||||||||||||||||||||||||||||||
Manhattan | E 84th St, E 85th St, 2nd Ave, 3rd Ave |
44
locations
|
||||||||||||||||||||||||||||||||||||||||||||||||
Queens | 38th St, Broadway, Steinway St, 34th Ave |
42
locations
|
If you’d like to explore other areas or type in your own NYC zip code, take a look at the accompanying map (be patient with her – she is large and slow):